Revolutionizing Data Annotation with Video Labeling Tools and Machine Learning
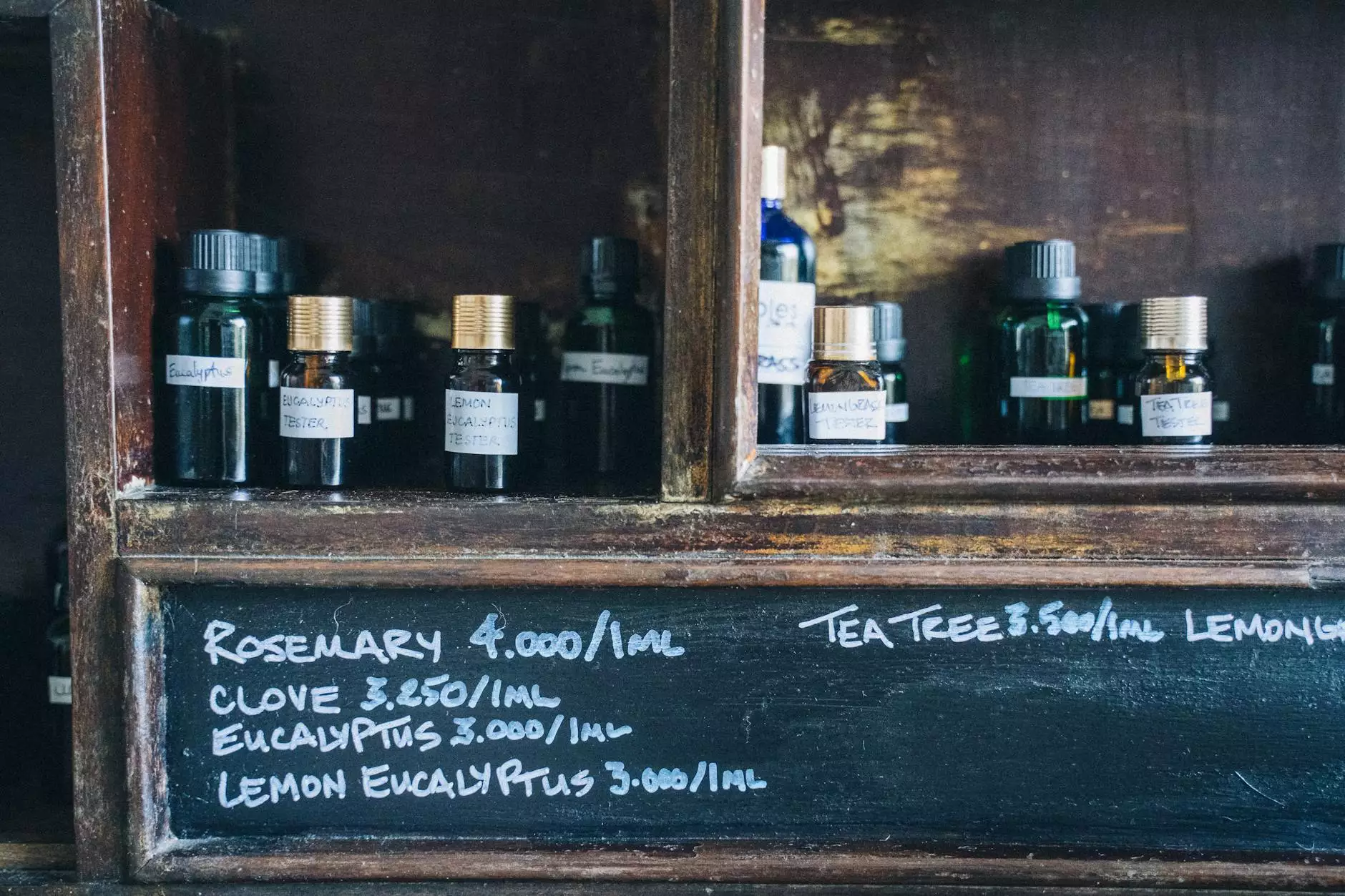
In the realm of data annotation, the convergence of video labeling tools and machine learning is reshaping how businesses approach data preparation and analysis. The necessity to process vast amounts of audiovisual data has propelled organizations to seek innovative solutions that enhance efficiency, precision, and scalability. This article delves deep into the dynamics of video labeling and the transformative power of machine learning technologies, showcasing how platforms like Keylabs.ai streamline this process.
The Importance of Video Labeling in Data Annotation
Video labeling refers to the meticulous process of identifying and annotating segments of video data. This can involve tasks such as:
- Image Classification: Identifying specific objects or actions within video frames.
- Object Tracking: Following movement of key subjects throughout the duration of the video.
- Action Recognition: Detecting and labeling specific actions performed by subjects in videos.
As machine learning models increasingly rely on annotated data for training, the precision and quality of video labeling become paramount. Business applications that utilize video data—ranging from autonomous vehicles to security systems—demand high-quality annotation to ensure effective model training and performance.
Challenges in Traditional Video Annotation Methods
While video annotation is crucial, traditional methods often face significant challenges:
- Time-Consuming Processes: Manual labeling is labor-intensive and can take days or even weeks to complete.
- Subjectivity and Inconsistency: Different annotators may interpret videos differently, leading to inconsistencies that can negatively impact machine learning outcomes.
- Scalability Issues: As the volume of data increases, scaling annotation efforts without sacrificing quality becomes a daunting task.
These challenges highlight the need for advanced solutions like machine learning-powered video labeling tools, enabling businesses to overcome traditional limitations and achieve superior results.
How Machine Learning Enhances Video Labeling
Machine learning introduces a paradigm shift in how video labeling is approached. Here’s how:
1. Automated Annotation
Machine learning algorithms can automate the video labeling process. By leveraging vast datasets, these algorithms learn to recognize patterns and make predictions, significantly reducing the time required for annotation.
2. Increased Accuracy
Through continuous training, machine learning models improve their accuracy in video annotation tasks. They can identify intricate details and multi-frame movements, which are often overlooked in manual labeling.
3. Real-Time Processing
Advanced video labeling tools can process video feeds in real-time, allowing businesses to annotate videos as they are being captured. This is particularly useful in applications like surveillance and traffic analysis.
4. Adaptability and Scalability
Machine learning models can be easily updated with new data, enhancing their ability to adapt to different types of video content without needing extensive retraining. This scalability makes them ideal for businesses facing rapidly changing data environments.
Key Features of Video Labeling Tools
Investing in a video labeling tool equipped with machine learning features offers numerous advantages. Here are some vital features to look for:
- Intuitive User Interface: A user-friendly interface ensures that both technical and non-technical users can engage with the tool efficiently.
- Batch Processing Capabilities: The ability to annotate multiple videos simultaneously saves time and resources.
- Integration with Machine Learning Frameworks: Seamless integration with popular machine learning frameworks enhances model training and deployment.
- Collaboration Features: Tools that allow multiple users to collaborate on projects can increase efficiency and consistency in data labeling.
- Quality Control Mechanisms: Automated quality checks ensure the reliability and accuracy of the annotations produced.
Applications of Video Labeling Tools in Various Industries
The applicability of video labeling tools powered by machine learning spans across multiple domains, influenced by the growing dependence on video data. Below are some examples of industries where these tools provide substantial benefits:
1. Autonomous Vehicles
In the automotive sector, self-driving cars leverage video data from cameras to navigate. Machine learning algorithms require extensively labeled training data to understand road signs, obstacles, and pedestrian movements accurately.
2. Healthcare
In healthcare, video analysis assists in monitoring patient behavior, physical therapy sessions, and even surgical procedures. Annotated videos allow practitioners to analyze techniques and refine skills effectively.
3. Security and Surveillance
Security systems utilize video feeds to detect anomalies and suspicious activities. Machine learning tools can quickly identify potential threats, enabling faster responses and enhancing overall safety.
4. Sports Analytics
Coaches and analysts use video labeling tools to break down game footage. Annotating plays can provide insights into player performance and strategizing for future games.
5. Retail
Video labeling helps retailers analyze consumer behavior in-store. By understanding how customers navigate space and interact with products, businesses can optimize store layouts and marketing strategies.
Choosing the Right Video Labeling Tool: What to Consider
Selecting a suitable video labeling tool is crucial for any business aiming to leverage machine learning effectively. Here are essential considerations:
1. Functionality and Features
Determine the specific labeling features needed for your projects, such as image segmentation or action classification, and ensure the tool meets those requirements.
2. Scalability
Your chosen tool should be able to scale according to your data needs. In a fast-paced environment, the ability to handle increased video input without sacrificing quality is vital.
3. Integration Capabilities
Consider how well the tool integrates with your existing systems, particularly machine learning frameworks and data storage solutions. Seamless integration can save time and improve output efficiency.
4. Cost-Effectiveness
Compare pricing models across different platforms. While some tools may require a high upfront investment, consider long-term value based on productivity gains and data accuracy.
5. Customer Support and Community
Robust customer support and an active user community can greatly enhance the user experience. Access to resources, troubleshooting, and collaborative problem-solving are significant advantages for any organization.
Future Trends in Video Labeling and Machine Learning
The future of video labeling seems promising with advancements in technology continually reshaping the landscape. Anticipated trends include:
1. Increased Utilization of AI
As AI technology evolves, video labeling tools will likely become even more sophisticated, requiring less human intervention in the annotation process.
2. Enhanced Collaboration
Expect to see more collaborative tools that enable teams to work together on video projects in real-time, regardless of location, promoting greater productivity.
3. Augmented Reality (AR) Integration
The integration of AR in video analysis could redefine how businesses interact with video informatics, enabling immersive experiences and more complex data visualization techniques.
4. Expansion into New Industries
As the understanding of machine learning’s potential grows, we will likely see wider adoption of video labeling tools in industries such as education, real estate, and environmental monitoring.
Conclusion: The Impact of Keylabs.ai on Video Labeling
In conclusion, video labeling tools powered by machine learning are revolutionizing the way businesses approach data annotation. By reducing manual labor, improving accuracy, and increasing scalability, companies like Keylabs.ai are at the forefront of this evolution. As organizations continue to harness the power of machine learning for data analysis and model development, the role of efficient and effective video labeling tools will only become more critical. Investing in these technologies is not just an option—it's a necessity for staying competitive in an increasingly data-driven world.
video labeling tool machine learning